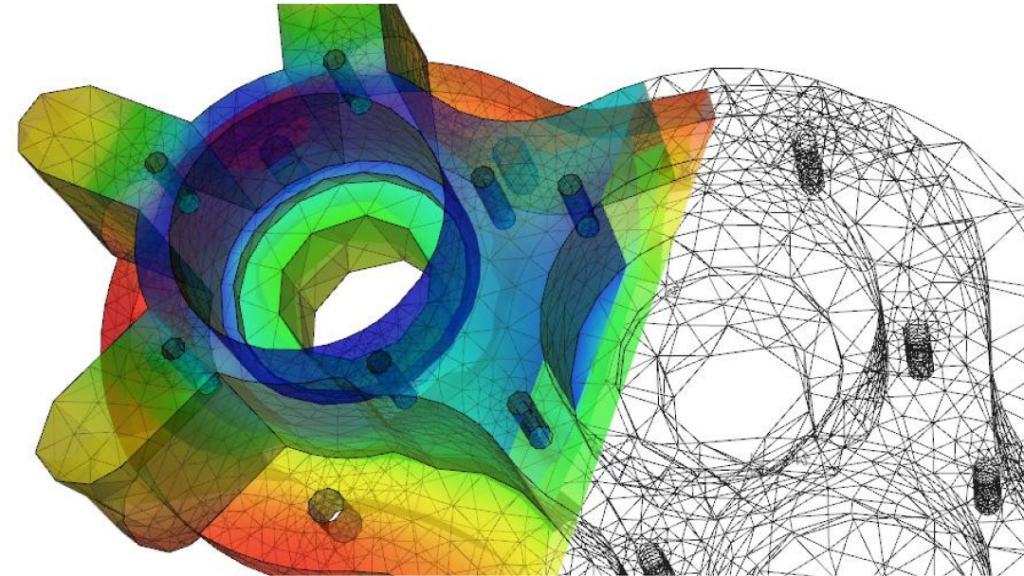
Engineering is undergoing a fundamental transformation as artificial intelligence systematically reconfigures how professionals think about conventional software development to computational design of physical systems. AI-augmented engineering assistants are spearheading this technical revolution, functioning as collaborators that mitigate repetitive elements which have historically consumed engineering cognitive bandwidth.
These AI-enabled systems have demonstrated significant efficacy in software development, technical documentation, and visual computing applications such as procedural image synthesis. However, implementation within the hardware development lifecycle remains substantially underexploited. At Cosmon, we believe that foundational AI architectures can be strategically deployed to address critical inefficiencies in computer-aided engineering (CAE) workflows. The discretization process—specifically the generation of finite element meshes—has traditionally represented a semi-heuristic procedure requiring iterative manual optimization. Contemporary machine learning systems now have the potential to generate simulation-ready discretizations by leveraging supervised learning from extensive datasets of validated mesh configurations, reducing pre-processing timeframes by approximately two orders of magnitude.
Simulation failure analysis, historically a resource-intensive diagnostic process, is being systematically optimized through AI systems capable of performing convergence analysis, identifying boundary condition instabilities, and recommending specific remediation protocols based on statistical pattern recognition across comprehensive simulation databases - perhaps even before simulations are run. This transforms stochastic troubleshooting procedures into deterministic, straightforward workflows.
Post-processing methodologies and crunching through large datasets are things that artificial intelligence excels at. In the future, engineers will be able to interface with CAE outputs through natural language queries such as: "Identify regions exceeding von Mises stress thresholds" or "Quantify the sensitivity of thermal gradients to material property variations." In addition to the engineer driving these questions, AI systems will performs multi-dimensional data analysis, extracts significant correlations, and generates appropriate visualization representations tailored to specific engineering analysis requirements.
Physics-informed neural networks (PINNs) also represent an adjacent advancement in computational simulation, fundamentally altering the speed at which you can approximate a differential equation. By encoding governing differential equations and boundary conditions directly within neural network architectures, these systems maintain physical conservation principles while providing computational acceleration by factors exceeding 100. Things like computational fluid dynamic simulations, previously requiring extensive high-performance computing resources, can now be executed on standard computational platforms, enabling rapid design space exploration and parametric optimization previously considered computationally prohibitive.
Despite these promising advances, significant challenges remain before AI can fully transform engineering simulation workflows. The accuracy of AI models in this domain remains a critical concern. While impressive in many scenarios, today's models still struggle with complex problems, showing limitations when faced with novel geometries or boundary conditions far from their training data.
Accuracy Imperative - The Engineering Standard
The consequences of even small errors in engineering applications can be substantial, making this accuracy gap a fundamental barrier to wider adoption. Unlike domains where approximate answers suffice, engineering simulation demands precision that many current AI approaches cannot consistently deliver, especially in safety-critical applications.
Trust and Verification - The Human-AI Partnership
Trust and the need for human oversight remain essential components of any AI-augmented engineering workflow. The "black box" nature of many AI approaches conflicts with engineers' need for explainable results that can be defended in high-stakes decisions about product safety and performance. Successful implementation requires human-in-the-loop systems where AI assists, but doesn't replace, engineering judgment.
Beyond Silver Bullets - Integration, Not Replacement
It's also important to recognize that there is no silver bullet replacement for traditional simulation methods. Different engineering problems demand different approaches—some are perfectly suited for AI acceleration, others requiring the precision of traditional solvers. The most effective systems will integrate both approaches, using AI where it excels and conventional methods where mathematical rigor is paramount.
Recognizing both the immense potential and unique challenges of AI in engineering simulation, Cosmon is building a custom foundation model platform specifically designed for computer-aided engineering applications. By training on diverse engineering datasets spanning structural mechanics, fluid dynamics, electromagnetics, and thermal analysis, Cosmon's models develop a deep understanding of physical behavior across domains.
Engineering Intelligence - Domain-Specific Foundation Models
Our platform creates an engineering-first, intuitive interface of an AI copilot for CAE, creating a system that speaks the language of engineers while respecting the underlying physics of the system under design. We're building a domain-specific CAE model trained on millions of engineering data points. More than just a language model, Cosmon represents a new approach to engineering simulation—one where AI amplifies human creativity and expertise rather than attempting to replace it.
The Collaborative Future - AI and Engineers Working Together
The future of engineering isn't about AI versus human engineers; it's about AI-driven engineers accomplishing what neither could achieve alone. Cosmon is proud to be building the foundation for this collaborative future, where AI-driven CAE becomes intuitive and reliable.